In a new article, experts in medical ethics outline their concerns with the use of machine learning and artificial intelligence (AI) in medicine and explore how the use of these algorithms may negatively impact care. Thomas Grote and Philipp Berens at the University of TĂźbingen, Germany, who study the ethics of machine learning in the medical field, wrote the article. It was published in the Journal of Medical Ethics.
Proponents of the use of artificial intelligence in the medical field suggest that machine-learning algorithms can be developed to process large amounts of data very quickly, and find patterns that clinicians might miss. While humans are prone to make mistakes under time pressure and given limited information, an algorithm could provide data that are more accurate. However, the researchers write, âthis narrative relies on shaky assumptions.â
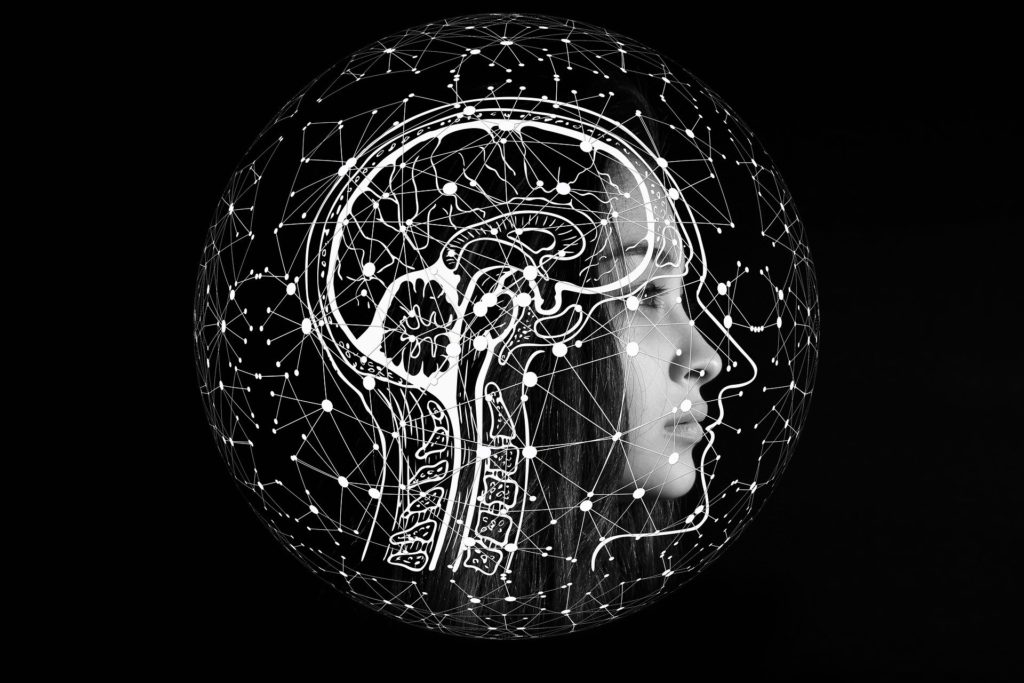
First, in terms of research, artificial intelligence algorithms are often found to equal clinicians in the ability to diagnose medical problems based on limited information in a short time frame. However, in real-life situations, the AI might not have access to the exact information it needsâand clinicians often have access to much more information than typically provided in these reports, such as the insight of a second clinician, other laboratory tests or images, and the report of patients. It is still unclear how well an algorithm would compare to clinicians in real-life situations.
The researchers identify a number of other issues with the use of machine learning. For one, they write that it âpromotes patterns of defensive decision-making which might come at the harm of patients.â If a clinician and the algorithm disagree, the computerâs report might be assumed more objective. Clinicians might also experience pressure from their employers to defer to the algorithmâs diagnosis, as that could be more defensible in court in cases of malpractice. This may be especially dangerous in extremely subjective cases such as the criteria for many mental health diagnoses.
Another issue is the âopacityâ of machine learning. Machine learning, by definition, is intended to come to conclusions that do not follow the normal patterns observed by humans. The algorithm is intended to detect patterns in large datasets that might be correlated with the diagnosis, and use those to make its decisions. It is often difficult for even the designers of such programs to tell how the AI came to its decisions, and it is even harder to explain that to a clinician or a patient. Whether to trust the algorithmâs decision may come down to having faith in its programming, which violates the rights of patients to have fully informed consent about diagnosis and treatment.
âAs the patient is not provided with sufficient information concerning the confidence of a given diagnosis or the rationale of a treatment prediction, she might not be well equipped to give her consent to treatment decisions.â
The researchers also discuss the potential for the algorithm to shift the normative standards for what is considered disease or risk of disease. Especially in the mental health field, the standard for what is a ânormalâ experience of suffering versus what is a âclinicalâ disorder may vary between health providers, communities, or regions of the world. An AI that has been trained to detect ADHD in children where it is over-diagnosed might go on to over-diagnose ADHD in another region, for instance. The line between âadjustment disorderâ and âmajor depressive disorderâ might hinge on whether the patient said a certain word or had a certain context for their experienceâbut an AI would have an almost impossible time distinguishing these factors.
Moreover, according to the researchers, because of the opacity of the algorithm, clinicians may never be able to tell whether this was the case or not. They would simply have to trust the algorithmâs decision.
Perhaps most concerningly, machine-learning algorithms usually reflect the biases of their creators. This should be of special concern in the psychiatric field, where diagnoses are often gendered or given more (or less) frequently to people of color.
According to a study published in Science, the very act of training artificial intelligence results in cultural biases being replicated in the AIâs output: âMachines learn what people know implicitly.â
Despite all these concerns, the researchers conclude:
âWe are convinced that machine learning provides plenty of opportunities to enhance decision-making in medicine. Medical decision-making involves high degrees of uncertainty and clinicians are prone to reasoning errors. In this respect, the involvement of machine learning algorithms in medical decision-making might yield better outcomes. However, it needs to be accompanied by ethical reflection.â
Despite broad claims of effectiveness, this year has been a difficult one for AI. In September, the app ImageNet Roulette, which routinely labeled pictures with racist and sexist statements, led to the deletion of half a million images from the ImageNet AI-training dataset. Meanwhile, researchers at UCLA and USC found that their AI repeatedly completed sentences about humans with racist, sexist, and homophobic responses.
Three of the main, large-scale AI-training datasets were deleted in July after an expose in Financial Times revealed that they consisted entirely of surveillance footage of American citizens taken without their consent and that they may have been used to train algorithms used by the foreign governments to track and imprison ethnic minorities. Then, in October, an AI project called DeepCom (led by Microsoftâs China office) was unveiledâan algorithm designed to create fake comments on news articles to boost engagement. Experts called it a vehicle for âtrolling and disinformation.â
****
Grote, T., & Berens, P. (2019). On the ethics of algorithmic decision-making in healthcare. Journal of Medical Ethics. Epub ahead of print. http://dx.doi.org/10.1136/medethics-2019-105586 (Link)
We can probably become more ridiculous.
Report comment
AI is probably useless for psych diagnosing, as psych “diagnoses” are totally subjective and inconsistent. With some decent data, AI diagnosticians could identify conditions that led to the alleged diagnoses, but incapable of telling shrinks anything about what they wanted to hear to direct their faulty prescribing.
Report comment
Have the AI been programmed with the reality that all the DSM disorders are scientifically “invalid” and “bullshit” yet? And that is according to the former head of NIMH, and the author of the DSM-IV.
https://www.nimh.nih.gov/about/directors/thomas-insel/blog/2013/transforming-diagnosis.shtml
https://www.wired.com/2010/12/ff_dsmv/
I doubt it, since the medical community is still utilizing the scientific fraud based psychiatric DSM “bible.”
The reality is our society needs to get back to the realization that medicine is still an art, not a science, particularly when it comes to psychiatry. And “It’s far more important to know what person the disease has than what disease the person has.” And certainly, medical AI programmed by deluded, racist, sexist, unethical, ignorant humans, is not a good idea.
“The line between ‘adjustment disorder’ and ‘major depressive disorder’ might hinge on whether the patient said a certain word or had a certain context for their experienceâbut an AI would have an almost impossible time distinguishing these factors.”
I have proof in my medical records that even the psychiatrists, themselves, can NOT tell the difference between ‘major depressive disorder,’ ‘bipolar,’ ‘schizophrenia,’ ‘adjustment disorder,’ or a healthy person dealing with a real life concern. Since I was misdiagnosed/defamed with all these “invalid” DSM disorders, so psychologists and psychiatrists could profiteer off of covering up the abuse of my child, according to all my family’s medical records.
Although, covering up child abuse and rape has apparently been, and still is, the number one actual societal function of both the psychologists and psychiatrists, for over a century.
https://www.indybay.org/newsitems/2019/01/23/18820633.php?fbclid=IwAR2-cgZPcEvbz7yFqMuUwneIuaqGleGiOzackY4N2sPeVXolwmEga5iKxdo
https://www.madinamerica.com/2016/04/heal-for-life/
Has the AI been programmed with the reality that the DSM does NOT allow ANY “mental health” worker to EVER bill ANY insurance company for EVER helping ANY child abuse survivor EVER, unless they first misdiagnose all abuse survivors with one of the billable DSM disorders?
https://www.psychologytoday.com/us/blog/your-child-does-not-have-bipolar-disorder/201402/dsm-5-and-child-neglect-and-abuse-1
At least humans eventually become embarrassed by their child abuse covering up crimes, but I doubt AI ever would.
Report comment
All Machine Learning algorithms are not created equal. Some algorithms are much more interpretable and lend themselves well in applications for highly regulated industries.
Atakan Cetinsoy
BigML – Machine Learning made easy and beautiful for everyone
Report comment
Atakante
And psychiatry is a big industry
Report comment