In a new article in JAMA, researchers suggest that developers of artificial intelligence (AI) programs for improving medicine should pay more attention to how their programs would actually function in a clinical setting. The authors remark that “given the abundance of algorithms, it is remarkable there has yet to be a major shift toward the use of AI for health care decision-making (clinical or operational).” However, they go on to outline several problems with AI programs that explain why healthcare organizations have not overwhelmingly adopted them for clinical decision making.
They write that “data quality, timeliness of data, lack of structure in the data, and lack of trust in the algorithmic black box are often mentioned as reasons.”
AI algorithms often haven’t been shown to work (“data quality”), nor have they consistently been found to improve results (due to “timeliness” or “lack of structure”). Another complication is that AI programs often can’t be assessed because their “black box” algorithms make it impossible to tell if they’re working, which leads to a “lack of trust.”
The authors acknowledge these problems, but they have an additional explanation, too: “Perhaps that model developers and data scientists pay little attention to how a well-performing model will be integrated into health care delivery.”
“The problem is that common approaches to deploying AI tools are not improving outcomes.”
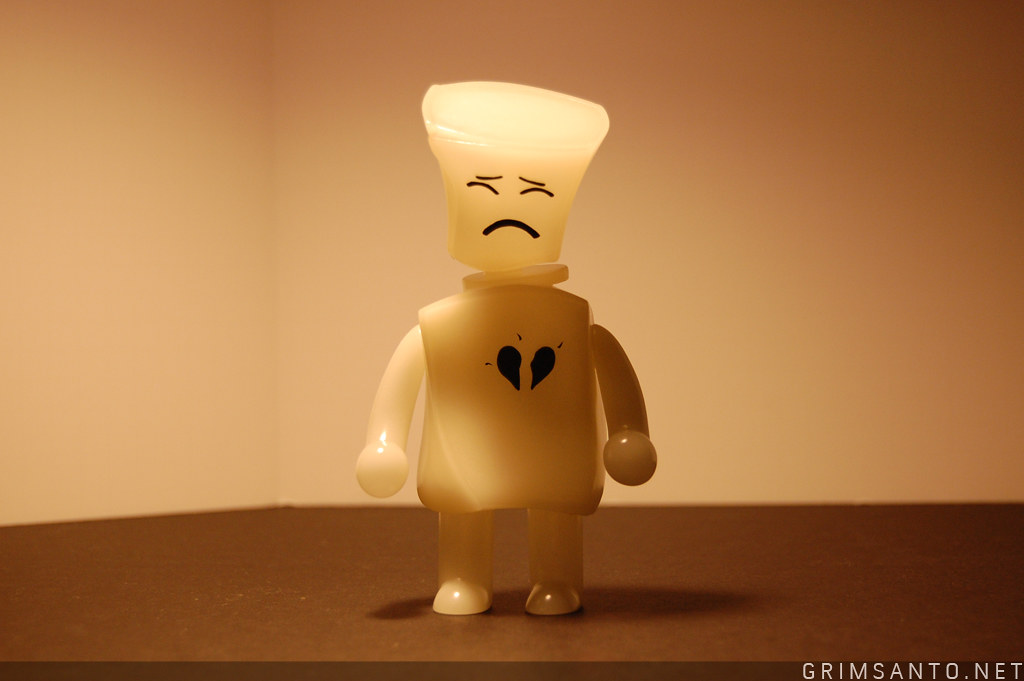
The lead author was Christopher J. Lindsell at Vanderbilt University Medical Center, himself a patent-holder on several predictive technologies who also receives funding from Endpoint Health Inc, an “early-stage” tech start-up in the healthcare field.
Lindsell and his co-authors suggest that one major problem is that even if artificial intelligence algorithms were shown to work, they may not improve outcomes. Importantly, prediction and surveillance do not necessarily improve healthcare outcomes.
“Designing a useful AI tool in health care should begin with asking what system change the AI tool is expected to precipitate. For example, simply predicting or knowing the risk of readmission does not result in decreased readmission rates; it is necessary to do something in response to the information.”
The authors suggest that technology companies should work with “end users” such as patients and clinicians to determine what algorithmic technology may actually be helpful—and “in some cases, the realization that the problem is not ready for an AI solution given a lack of evidence-based intervention strategies to affect the outcome.”
They provide an example of an “expensive intervention” aimed at reducing alcohol use in people who experienced trauma. Technically, the intervention worked—but only for the people who were at low risk of both alcohol use and readmission.
“It was ineffective for those with more serious alcohol-related problems, who are also at higher risk of readmission.”
So, in that instance, a technology was developed with good intentions, and it appeared at first glance to be successful. But upon further review, it actually failed to work for the group of people who needed it most.
Apps using artificial intelligence to assess mental health are already in use, partnering with health insurance companies and medical centers, despite no published research evidence demonstrating their effectiveness in any clinical domain.
There were over 325,000 different healthcare apps available to download in 2017, and the market share was estimated at $23 billion. In 2018, users downloaded over 400 million healthcare apps, and that number has likely only grown.
A study last year found that of the more than 10,000 apps available for mental health, only “3.41% of apps had research to justify their claims of effectiveness, with the majority of that research undertaken by those involved in the development of the app.”
****
Lindsell, C. J., Stead, W. W., & Johnson, K. B. (2020). Action-informed artificial intelligence—Matching the algorithm to the problem. JAMA. Published online May 1, 2020. doi:10.1001/jama.2020.5035 (Link)
There’s no such thing as an “app deficit” in human medicine….that’s a business and financial problem. ALL problems in medicine are HUMAN, not AI. And the biggest problem with AI is the so-called “AI Virus….. I think in future years we’ll see few of the alleged benfits of AI pan out….
Report comment
Well when you consider how so many shrinks aspire to become AI, it seems you could easily replace them with software apps and do just as well.
Report comment
Or better. At least software apps can’t be specifically prejudiced against a particular person.
Report comment
Ah but they can. That’s called “the algorithm. It has been shown that algorithm get way skewed and prejudiced – the prejudices of the people writing the parameters get amplified in the echo chambers of algorithms and turn into very prejudicial AI’s….
Though – you said *one* person. It would depend on what parameters on the algorithm any one person fits or doesn’t fit.
Report comment
Well, of course, the algorithm is only as good as the programmer. I’m sure someone could program a discriminatory app. But at least they won’t have to manage their emotional reactions to our statements, appearance, etc. I’m sure they’d totally suck, because they’d be made by people who have no comprehension of what is helpful, otherwise, they’d realize that a computer can’t provide what is needed.
Report comment
Absolutely! Algorithms are not neutral; they reflect the biases of those who create them. When designed without careful oversight, they can amplify prejudices, reinforcing echo chambers and skewed perspectives.
That said, whether one person gets affected depends entirely on how they align with the algorithm’s parameters. Some may slip through unnoticed, while others may find themselves disproportionately impacted. The key issue isn’t just the algorithm itself but how its design shapes outcomes—intentionally or unintentionally.
Report comment
The data quality issue is largely of the medical field’s own making. Format and contents of clinical documentation are determined by insurers, governmental regulatory officials, and other funding sources. Data is generally only collected for the purpose of billing and not for future clinical use or research/training ML algorithms. I’m not sure what they mean by ‘black box’ either. Just because YOU don’t understand the math, doesn’t mean I can’t. Backprop is fundamental to how neural networks learn from data so you should always be able to follow backwards through the model from the decision in the output to the data in the input. The real problem is the lack of collaboration between the two fields. I don’t see the example above as a failed intervention. AI was able to cheaply address the low risk population, leaving the experts more time to focus on the ‘problem children’. That’s a failure of the treatment program to utilize the treatment in the best way possible. Remember, AI based cancer diagnosis only beats human based diagnoses slightly. The real improvement comes from augmenting the human. Human’s armed with Ai diagnostic data perform better than any ML algorithm ever will.
Report comment
“The problem is that common approaches to deploying AI tools are not improving outcomes.”
No, the problem is that common approaches to “helping” with mental/emotional/spiritual distress don’t improve outcomes, and no amount of AI is going to change the fact that the basic model of distress and helping is fatally flawed. Well, flawed unless your “outcome” is increased profits. Maybe that’s what they mean – AI isn’t improving income, therefore, it isn’t working?
Report comment
The outcomes for whom? The Human or the Institutes of Technology? Any knowledge of what drives the Broad Institute on the MIT campus? How does one create breathing space for anyone, students & professors to ask questions of each other without being told, to soon for lack of deep listening, or how to listen to what is attempting to be asked, without thinking of an Oedipus sort of destructive mentality that often emerges in therapy, if one challenges the ruler in the sessions? Or are the “Orders” in the challenge to become a knowledge producing economy undermining authentic health? And care?
Report comment